APPAM founded the Journal of Policy Analysis and Management (JPAM) in 1981 through the merger of two other journals – Policy Analysis and Public Policy. Wiley-Blackwell (formerly John Wiley & Sons) has published JPAM since 1981. JPAM is published quarterly and is a peer-reviewed research journal. APPAM Members can access the online version of JPAM via their membership page.
JPAM's Closer Look, the podcast featuring interviews with authors, and JPAM At a Glance, which distills select articles into bite-sized summaries, are available to all. Learn more below.
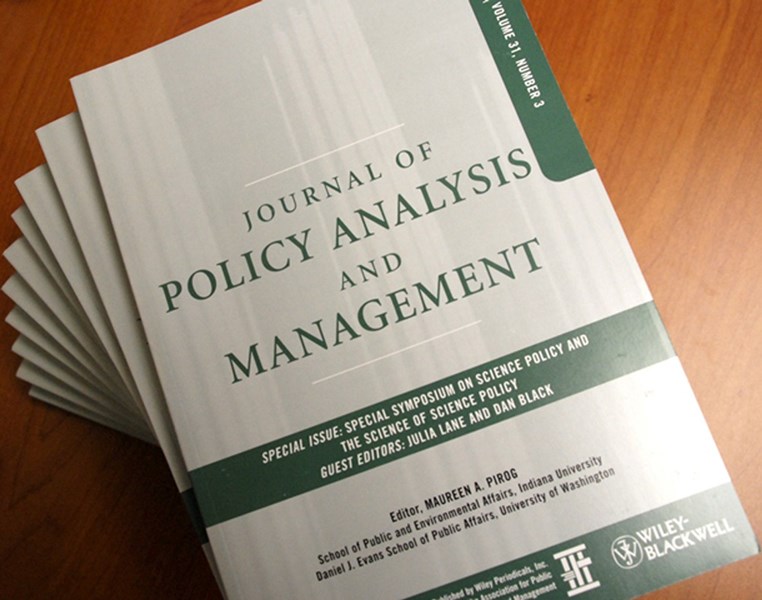
The creation of JPAM fulfilled one of the primary reasons for APPAM's existence: the dissemination of the highest quality multidisciplinary research in public policy and management. As the Association's journal of record, JPAM's ultimate purpose is building a professional community of scholars and practitioners devoted to more effective policy analysis and public management. JPAM welcomes unsolicited manuscripts from all sources. For more information on submitting to JPAM, click here.
JPAM is one of the leading journals in the field of public policy and public management. Based on the 2018 Journal Citations Report impact factor, it is ranked among the top four journals of public administration. In the same year, JPAM was ranked 28th out of 363 journals in the economics category.
In 2018, readers made over 158,000 downloads of articles. Topics of the most commonly downloaded articles cover a wide range of interests: The effects of paid family leave in California on the labor market, the impact of the No Child Left Behind legislation, the Kyoto Protocol and carbon emissions, the decriminalization of marijuana, the persistence of poverty in the context of financial instability, the significance of child-care subsidies on child development, and the policy implications of food deserts in the United States.
Each year, JPAM honors three reviewers at all career stages who have demonstrated excellence in terms of quality and efficiency of their refereeing for the journal. Learn more about the JPAM Refereeing Award here.
JPAM is under the management of its editor, appointed to a five-year term by the APPAM Policy Council. The editors to date:
The JPAM editor works with an associate and section editors and editorial board appointed to fixed, staggered terms. The editorial board advises the editor on matters of policy. Every year APPAM awards the Raymond Vernon Memorial Prize for the best article published in the current volume of JPAM. The prize selection committee usually is drawn from the editorial board, and Wiley underwrites all expenses related to the prize.
The JPAM editor also appoints section editors to manage five special sections in the journal: Point/Counterpoint, Methods for Policy Analysis, Policy Insights, Policy Retrospectives, and Book Reviews. These sections usually publish shorter, specialized articles on those topics.
JPAM is available online-only and no longer produces a print edition.
To access JPAM articles online:
- Login to your APPAM account by clicking the Login button on the top of the APPAM website. You will be directed to the Member Landing Page.
- On the Member Landing Page, select JPAM Online Access from the Quick Links sidebar on the right side of your screen.
- You will now be directed to a page with a temporary JPAM access link. Click on the text that says "Click here to access the journal."
- You should now be redirected to JPAM's online home via Wiley, with full access to each article.
Click here for a link to this guide featuring images of where to click, troubleshooting tips, and a downloadable PDF of the instructions.
JPAM also produces a public policy interview podcast, JPAM's Closer Look.
JPAM at a Glance offers a bite-sized way to communicate the main points of the articles in JPAM.
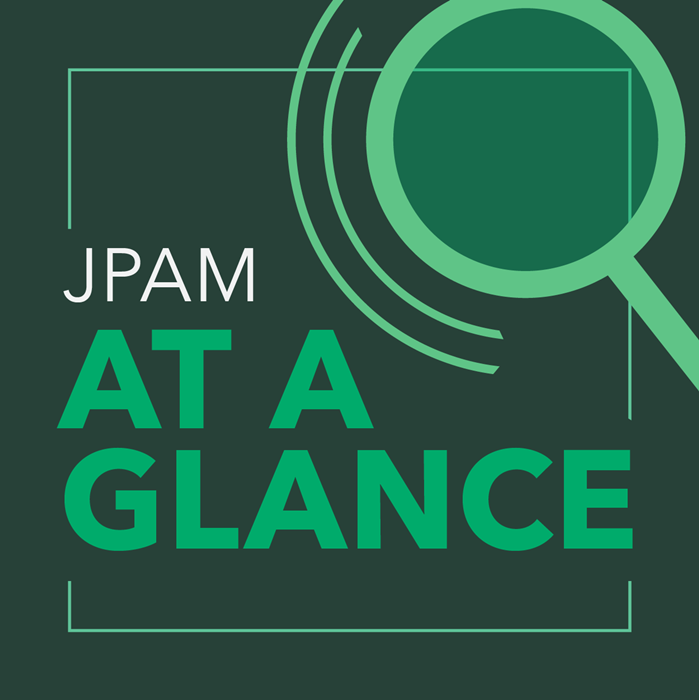
Browse previous editions: